In today's conditions of stiffer competition, it is not so easy to ensure the profitability and commercial success of an enterprise, especially a high-tech one. The most profitable way to acquire significant competitive advantages is to continuously improve the quality of the product, rather than a permanent reduction in costs. Artificial intelligence will help to cope with this task. With its help it is possible to significantly simplify the management of technological processes, and for the implementation it will not require a radical restructuring of existing production.
A quality control system based on the work of self-learning neural networks can simultaneously track a large number of parameters and analyze their slightest changes.High-tech developments make it possible to make expert decisions in a fraction of a second, increasing the accuracy of control actions and, as a result, reducing the number of manufacturing defects. Due to this, quality control organization becomes as efficient as possible.
In addition to high production speed, automation of technological processes makes it possible to maximize the use of personnel reserves. The product quality management system can be subordinated to one operator. Due to this, specialists of a higher profile have more opportunities to assess the state of production and optimize the entire production process.
Thus, the automatic quality control of products is easy to use, allows you to reduce costs and increases the overall speed of the enterprise. Artificial intelligence can cope with large amounts of information and the results of its analysis will be objective and impartial. The result of the implementation is high productivity and competitiveness of enterprises, which is especially important in complex markets.
The digitization of an increasing number of production processes has a positive effect on the work efficiency, profitability of the enterprise, and product quality. Artificial intelligence is able to carry out sound and productive management of technological processes, due to which there is a more rational distribution of available resources. The very increase in production efficiency is carried out on the basis of the fact that, using digital solutions, an enterprise becomes more flexible and adaptive to rapidly changing competitive conditions, and all the processes inside it are much faster. Proper distribution of tasks between Artificial Intelligence and Man makes it possible to reduce the number of engineering and technical specialists, without losing as a product.
However, reducing production costs is impossible in the long term without the introduction of reliable and high-quality intelligent systems.
It is important that the automation of technological processes was carried out taking into account the specific specifics of the enterprise and the products sold.This condition is one of the key to success. For this reason, more and more often, the reduction of production costs is not carried out on the basis of ready-made template solutions. It requires an individual approach, the development and implementation of unique intelligent systems that would fully meet the requirements of a particular production. Modern scientific and technical developments offer a wide range of tools and solutions for tracking control at all stages of ensuring security, trouble-free and uninterrupted operation of equipment. They can be successfully combined with each other, refined and modernized, so that the cost reduction occurs as quickly and efficiently as possible even for complex industries.
Production management in a market economy, when the numerous risks of an enterprise are sometimes difficult to classify, make it necessary to use various methods and computational programs to make the most accurate and informed decisions. For management to be effective, it is important not only to choose the most rational strategy, but also to select a set of applied tools that will reduce the time and labor costs for solving managerial tasks.
Methods of forecasting and calculating the risk of marriage of finished products
Modern systems of artificial intelligence allow you to automatically predict the quality of products and assess the likelihood of the risk of a marriage. Our team has developed an artificial intelligence system - CING, which continuously analyzes data from sensors and measuring equipment. Through the use of diverse metrics and evaluation of equipment operation trends, the system provides the ability to predict with high accuracy the result of the technological process, to its actual completion, and to make the necessary adjustments automatically to improve the final quality of the product.
Such an analysis becomes possible through the use of modern neural network algorithms of artificial intelligence system CING.
In real-time, the system collects information from various sensors and assesses the quality of the process flow, after which it provides data in the form of information about the expected quality and the likely risk of a finished product.The use of artificial intelligence in this case improves the awareness of the process, reduces the likelihood of human error, and increases the ability of staff to make timely adjustments to achieve maximum efficiency of the process.
Automated process control is a cost-effective solution for enterprises. The forecasting and calculation of production risks carried out in this way can reduce internal costs, as well as reduce the labor costs of collecting and analyzing all the necessary data. As a result, the company receives a minimum share of manufacturing defects, reduced operating costs, reduced downtime and the number of emergency cases in the absence of continuous monitoring by individual specialists.
Today for modern production one of the primary tasks is the control of industrial safety equipment. Entire complexes of complex systems, special equipment for improper handling and untimely troubleshooting are a serious source of risk for workers and the environment. This is especially true for complex productions.
No less dangerous is the uncertainty that hides the work of complex equipment without collecting complete and reliable information about the state of individual systems.
To prevent various breakdowns, reduce the risk of accidents, more and more often technological processes are controlled using intelligent digital systems.This allows not only to increase the reliability and diversity of the information received, but also to reduce the labor costs of production. Today, automation of technological processes is a reliable way to ensure high efficiency and safety of production, as well as high quality of manufactured products.
The practical benefits of control with the involvement of neural networks
Industrial safety systems of equipment, based on artificial intelligence, independently solve a whole range of tasks that previously required the presence of individual specialists. These include:
- failure analysis;
- diagnostics of existing and possible malfunctions;
- efficient collection of all required data from various sensors and devices;
- processing the information received;
- making management decisions at the expert level of rationality and efficiency.
The CING hot rolling process forecasting system is a software product based on a mathematical model developed using machine learning methods that calculate and predict the following operating parameters of the hot-rolling mill equipment:
The main functions of the system.
The system has a modular structure. This system includes the following features:
- Predicting roll wear and peak loads;
- Calculation of the presets of the rolls of the hot-rolled mill;
- Wide rolling to narrow planning;
- Prediction of slab outlet temperature.
- Calculation of the speed of the roller table to achieve a predetermined temperature (at the intermediate cooling and cooling installation);
- Obtaining parameters of the measured band from the upper level system (Steel grade, tasks ...)
- Visualization of input and calculation data
- Archiving input and calculation data
- Indication of incorrect operation of sensors
- Accounting for the effect of steel grade on the design model
The system is based on a mathematical model. It is based on artificial neural networks, but is not limited to them.
A distinctive feature of neural networks is their ability to learn - that is, to increase their own efficiency based on well-known examples. Therefore, to create a neural network, you must have data with a known result - the so-called training with a teacher.By learning from the available data, the neural network achieves the required accuracy, after which it can be used as a model for calculating parameters in real time.
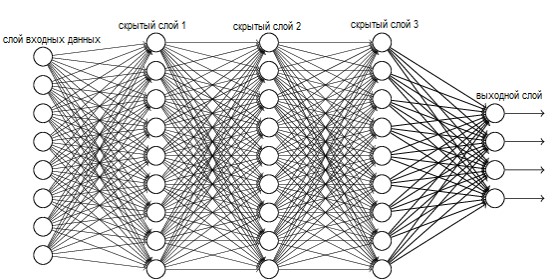
Pict.2. Multilayer neural network architecture
The CING system provides for preliminary training of the calculation modules at these enterprises. These modules will later receive the necessary data from the top-level system and carry out the calculation of target information, after which this information is archived and access to its analysis is provided.